Home » Simplifying Middle Office and Back Office workflows with STP and Automation
INSIGHTS
Intelligent solutions. Informed decisions. Unrivaled results.
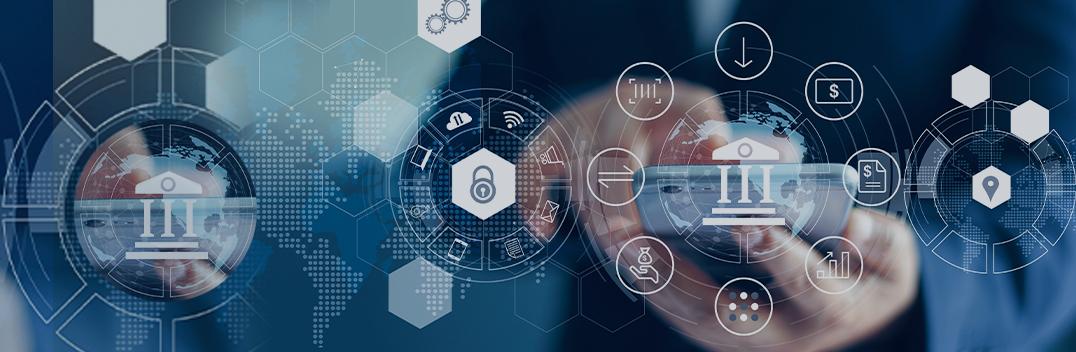
Simplifying Middle Office and Back Office workflows with STP and Automation
The Banking and Financial Services Sector and the fintechs supporting them have not been unaffected by the winds of change sweeping across the business landscape. But unlike the past, technology has proved to be an equalizer. Size is no longer a necessary condition for success. Today we have Challenger Organizations, typically SMEs and large institutions alike, competing on a level playing field, and whosoever simplifies their processes or automates first gains an edge. Examples of how Intuit, Square, and similar Challenger Organizations redefined the meaning of Customer experience are proof enough. Automation, elimination of repetitive manual tasks, and consolidation of redundant activities into fewer steps have played a crucial role in enhancing Straight Through Processing (STP).
Straight Through Processing has hitherto been addressed by optimizing underlying applications, eliminating data silos, and integrating applications better. While process automation through RPA and similar technologies have helped optimize downstream processes, the manual effort was still significant due to the disparate data sources that could not be consolidated and integrated. It is this final boundary that is sought to be breached through the application of Emerging Technologies. With a holistic end-to-end straight-through processing (STP), banks and FIs have taken a quantum leap forward and what would have once taken days to accomplish now takes minutes. STP removes the need for manual intervention. The only time human intervention is “ ideally” required is during “exception handling ” or “exceptions processing” - when the system sees something that is unusual and raises a red flag. The human annotator then sets about making the necessary changes.
White whales for STP implementation or Taming the white whales (with STP)
STP implementation is ideal for processes that involve a lot of repetitive work. The costs of system integration (might) dissuade many smaller players from considering it. However, like Captain Ahab’s relentless pursuit of the White Whale - Moby Dick, digital transformation experts have relentlessly argued the case for STP implementation (without similar calamitous consequences) in some of the most tiresome and time-consuming processes like KYC, loans processing, foreign exchange transaction handling, accounts payables amongst others. Organizations that must meet quality SLAs as part of the business agreement have much to lose if they do not innovate on the technology front. Humans are more liable to make errors - attach a wrong document, classify a document incorrectly (highly probable if there are 100 odd classifications to choose from), or simply feed data incorrectly into the system. And in the event this takes place, the likelihood of not meeting the SLAs (resulting in client dissatisfaction) is high.
Secondly, banks and FIs, manually administering processes like KYC, have no time for value-added activity (like sales and customer retention and experience) as they are busy meeting the deadlines. As manual labor is both slow and expensive, there is a considerable backlog. Let’s take the example of the KYC process - a McKinsey report states that banks generally employ about 10 percent of the workforce in financial-crime-related activities. Now that is a lot in terms of labor cost. The report has also indicated that KYC reviews were often the costliest activities handled by the bank.
With STP, banks and the financial services sector can eliminate a lot of paperwork, many unnecessary checkpoints (translating into unnecessary headcount), manual data entry while ensuring that SLAs are met and invoices, KYC, onboarding, accounts payable and accounts receivable, and other such processes and document-oriented activities are conducted quickly and cost-efficiently and with relatively fewer margins of error. So that organizations can ensure higher levels of transparency and trust and a good customer experience.
Cost of quality
Let’s be honest; even the best human keyers/classifiers make more errors or mistakes than machines. While an error percentage of 2 to 5 % might not seem much, if we apply the 1-10-100 rule for the “cost of quality” and take into account a million documents that are being classified and whose data is being extracted, 5 % does make a huge difference. Automatically that would translate into a lot of work that would require human intervention. For every error that could be prevented, the cost of rectifying it is 10 times more. Leaving an error unattended is costlier - 100 times more expensive than doing it right the first time.
Machines, however, are more capable. A Mckinsey report states, “ Assuming that standardization and coding of rules are performed correctly, quality can be improved significantly (by a range of 15 to 40 percent, experience indicates). Manual errors are reduced, and the identification and documentation of risks are improved. Rework loops can be shortened as well, as “first time right” ratios and regulatory targets are met more quickly.”
And now comes the really tricky part, since 100 percent accuracy is still unthinkable even for machines. When we are talking about documents, and relevant data fields, an accuracy of 99 % at the character level does not ensure STP. If documents need validation from their human supervisors due to high error rates, zero STP will ensue. Here we’d need something more robust than RPA. With machine learning (ML) and advanced capture, it is possible to increase accuracy to validate data using advanced rules. We’d need a system that constantly adjusts and optimizes data. So every time the system encounters a variance or an anomaly, it adapts ( taking help from the human-in-loop) and improvises, becoming better after each iteration.
We would also have to take into account the variance in straight-through processing when it comes to structured and unstructured documents. While a standardized document such as a 10k will enable higher levels of STP, semi-standardized documents such as invoices and unstructured documents such as notes and agreements will allow lower levels of STP.
Simplifying banking processes
Today the imperative for banks and FIs to simplify their processes is huge. Future growth today is dependent on the ease with which banks and FIs can conduct their business. There is no escaping that! Reiterating the need for simplification, Hessel Veerbek, partner strategy, KPMG Australia, writes about “how some banks and insurers have replaced key elements of their core systems and consolidated their ancillary systems to rationalize their IT estate, modernize their capabilities, reduce costs and, at the same time, provide the capabilities to adapt and evolve their business models to secure future growth.”
Banks need to simplify operation by a core banking system that takes care of processes like loans processing and accounts payable end-to-end. At the heart of a simplified and automated banking architecture is end-to-end STP, which can be a complex undertaking, but as leading banks have shown, it is worth the trouble as it boosts efficiency. The challenges to STP incorporation are really in the mindset of organizations as “complex processes, high-risk customers and non-standard accounts” are still excluded from the purview of STP. It’s typical for organizations to consider STP while conducting low-risk tasks such as KYC for low-risk accounts in the KYC process. However, as the Mckinsey report suggests, if applied with dexterity and foresight, STP can eventually be enabled for the high-risk segment of customers as well. But here, a simple rules-based approach will not suffice, and organizations would need to rely on data science to create a system that will ensure a reliable output.
Augmenting human labor - when machine learning tools perform the task and optimize it as well
The question is not really about how STP reduces the need for manual intervention - it is about how it augments human skills. The time it takes a human to classify and extract information from documents is disproportionate to the gains. Considering that almost 100 % of all data entry tasks can be automated and results can be obtained in a fraction of the time, it makes sense to invest in tools that would ensure end-to-end automation. With STP, banks and Fintechs can not only eliminate the need for manual keying and classification of data, but in time sophisticated machine learning tools can also eliminate the need to verify that data manually.
Getting to zero-touch!
For true STP, we want an error rate that is such that human intervention is not required. This STP is the percentage or number of documents that go through the system with zero-touch or human contact. If the error rate or adverse media reaction is high, every document would have to be reviewed. Hence organizations must work on increasing accuracy by leveraging the power of AI and ML tools.
If we are talking about cost efficiency, the need for software that easily integrates with organization-wide legacy systems is also a prerequisite.
The automation success story is not only about STP.
It is important to remember that the automation success story does not depend on STP alone; other factors like investment costs, capital performance, cycle time, ROI, headcount reduction also matter. While “customer satisfaction” and “experience “ are good to have, Net Present Value (NPV), cost efficiency, and headcount reductions matter a lot. After all, leaner, nimbler, and more efficient operations are what most organizations are after.
While considering STP, it is also essential to do some homework regarding the investment costs, the complexity of the process (number of data elements that must be extracted, variance in documents, etc. ), cycle time, and the headcount reduction, etc.
Experience tells us that the shift from highly manual-oriented processes to STP is not easy. It requires massive levels of patience and commitment as it takes time to reach the desired levels of accuracy. The actual test of STP success for any process depends on determining with a high degree of precision if a task has been executed accurately or not. A high rate of error or human intervention results in zero STP.
Regardless of the challenges underlined earlier, STP remains a significant milestone in any organization’s journey towards automation. Banks and FIs that have successfully implemented STP have reaped many visible benefits. With Straight Through Processing, banks and FIs can choose to re-direct their efforts towards customer experience and retention, as they now have the time and bandwidth. When banks and FIs automate invoices and payments, they pave the way for a happier customer and employee experience.
The question today is not whether STP is the ultimate test for automation progression; the question today is whether organizations can afford to do without STP - considering the astronomical costs of processing files and increased competition. Magic FinServ, with its years of experience serving a diverse clientele set comprising some of the top American banks and Fintechs, is well acquainted with the opportunities and risks associated with process optimization and simplification using AI and ML. If organizations are not careful, the costs could escalate disproportionately and disrupt the drive towards digital transformation. Magic FinServ helps you navigate uncharted waters by leveraging our understanding of the financial services business to re-engineer existing applications, design new platforms, and validate machine learning solutions to suit your business needs. To explore our solutions, reach out to us mail@magicfinserv.com