Home » Accelerate Financial Forecasting through Data Consolidation and Data Maturity before recommendation Engines
INSIGHTS
Intelligent solutions. Informed decisions. Unrivaled results.
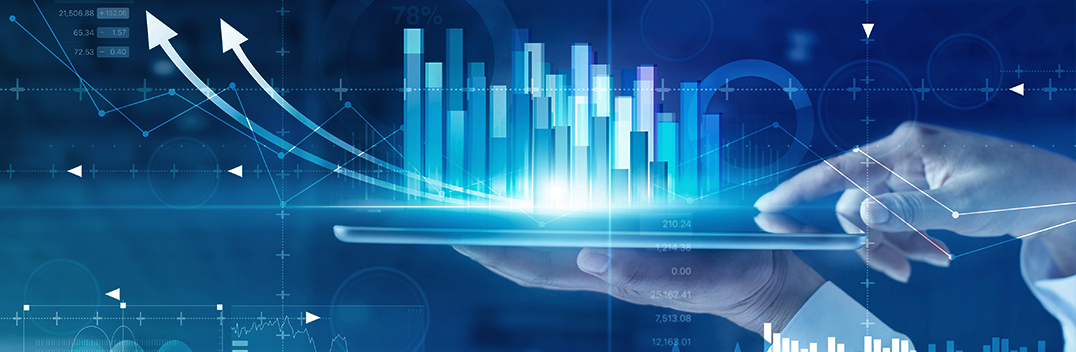
Accelerate Financial Forecasting through Data Consolidation and Data Maturity before recommendation Engines
Jim Cramer famously predicted, "Bear Stearns is fine. Do not take your money out. "
He said this on an episode of Mad Money on 11 March 2008.
The stock was then trading at $62 per share.
Five days later, on 16 March 2008, Bear Stearns collapsed. JPMorgan bailed the bank out for a paltry $2 per share.
This collapse was one of the biggest financial debacles in American history. Surprisingly nobody saw it coming (except Peter, who voiced his concerns in the now infamous Mad Money episode). Sold at a fraction of what it was worth – from $20 billion capitalization to all-stock deal values of $ 236 million, approximately 1% of what it was worth earlier, there are many lessons from the Bear Stearns fall from grace.
Learnings from Bear Stearns and Lehman Brothers debacle
Bear Stearns did not fold up in a day. Sadly, the build-up to the catastrophic event began much earlier in 2007. But no one heeded the warning signs. Not the Bear Stearns Fund Managers, not Jim Cramer.
Had the Bear Stearns Fund Managers ensured ample liquidity to cover their debt obligations; had they been a little careful and understood and accurately been able to predict how the subprime bond market would behave under extreme circumstances as homeowner delinquencies increased; they would have saved the company from being sold for a pittance.
Or this and indeed the entire economic crisis of 2008, was the rarest of rare events, beyond the scope of human prediction – a Black Swan event, an event characterized by rarity, extreme impact, and retrospective predictability. (Nassim Nicholas Taleb)
What are the chances of the occurrence of another Black Swan event now that powerful recommendation engines, predictive analytics algorithms, and AI and ML parse through data?
In 2008, the cloud was still in its infancy.
Today, cloud computing is a powerful technology with an infinite capacity to make information available and accessible to all.
Not just the cloud, financial organizations are using powerful recommendation engines and analytical models for predicting the market tailwinds. Hence, the likelihood of a Black Swan event like the fall of Bear Stearns and Lehman Brothers seems remote or distant.
But faulty predictions and errors of judgment are not impossible.
Given the human preoccupation with minutiae, instead of possible significant large deviations, even when it is out there like an eyesore, black swan events are possible (the Ukraine war and subsequent disruption of the supply chain were all unthinkable before the pandemic).
Hence the focus on acing the data game.
Focus on data (structured and unstructured) before analytics and recommendation engines
- The focus is on staying sharp with data - structured and unstructured.
- Also, the focal point should be on aggregating and consolidating data and ensuring high-level data maturity.
- Ensuring availability and accessibility of the "right" or clean data.
- Feeding the "right" data into the powerful AI, ML, and NLP-powered engines.
- Using analytics tools and AI and ML for better quality data.
Data Governance and maturity
Ultimately financial forecasting - traditional or rolling is all about data from annual reports, 10-K reports, financial reports, emails, online transactions, contracts, and financials. As a financial institution, you must ensure high-level data maturity and governance within the organization. For eliciting that kind of change, you must first build a robust data foundation for financial processes, as advanced algorithmic models or analytics tools that organizations use for prediction and forecasting require high-quality data.
Garbage in would only result in Garbage out.
Consolidating data – Creating a Single Source of Truth
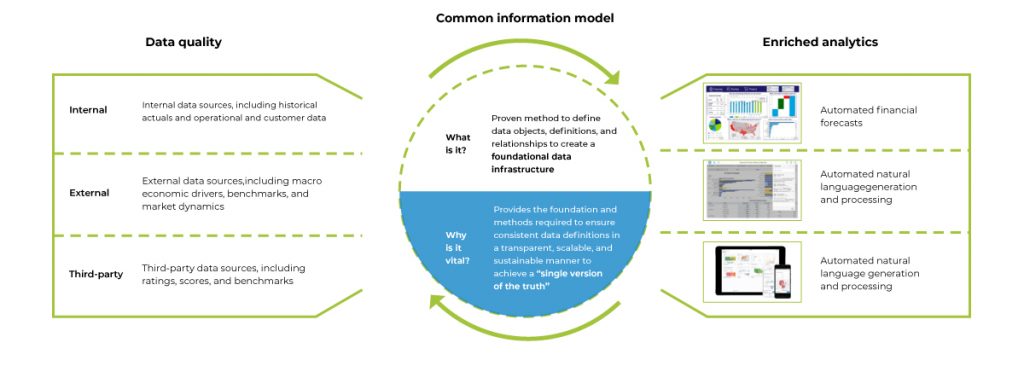
Source: Deloitte
- The data used for financial forecasting comes primarily from three sources:
- Data embedded within the organization - historical data, customer data, alternative data - or data from emails and operational processes
- External: external sources and benchmarks and market dynamics
- Third-party data: from ratings, scores, and benchmarks
- This data must be clean and high-quality to ensure accurate results downstream.
- Collecting data from all the disparate sources, cleaning it up, and keeping it in a single location, such as a cloud data warehouse or lake house - or ensuring a single source of truth for integration with downstream elements.
- As underlined earlier, bad-quality data impairs the learning of even the most powerful of recommendation engines, and a robust data management strategy is a must.
- Analytics capabilities are enhanced when data is categorized, named, tagged, and managed
- Collating data from different sources - this is what it was and what is - historical trend analysis.
Opportunities lost and penalties incurred when data is not of high quality or consolidated
Liquidity assumption:
As an investment house, manager, or custodian, it is mandatory to maintain a certain level of liquidity for regulatory compliance. However, due to the lack of data, lack of consolidated data, or lack of analytics and forecasting, organizations end up making assumptions for liquidity.
Let's take the example of a bank that uses multiple systems for different portfolio segments or asset classes. Now consider a scenario where these systems are not integrated. What happens? As the organization fails to get a holistic view of the current position, they just assume the liquidity requirements. Sometimes they end up placing more money than required for liquidity, which results in the opportunity being lost. Other times, they place less money and become liable for penalties.
If we combine the costs of the opportunity lost and the penalties, the organization would have been better off investing in better data management and analytics.
Net Asset Value (NAV) estimation:
Now let's consider another scenario - NAV estimation. Net Asset Value is the net value of an investment fund's assets less its liabilities. NAV is the price at which the shares of the funds registered with the U.S. Securities and Exchange Commission (SEC) are traded. For calculation of month-end NAV, the organization would require the sum of all expenses. Unfortunately, as all the expenses incurred are not declared on time, only a NAV estimate is provided. Later, after a month or two, once all the inputs regarding expenses are made available, the organization restates the NAV. This is not only embarrassing for the organization as they have to issue a lengthy explanation of what went wrong but are also liable for penalties. Not to mention the loss of credibility when investors lose money as the share price is incorrectly stated.
DCAM Strategy and DeepSightTM Strategy – making up for lost time
Even today, when we have extremely intelligent new age technologies at our disposal - incorrect predictions are not unusual. Largely because large swathes of data are extremely difficult to process - especially if you aim to do it manually or lack data maturity or have not invested in robust data governance practices.
But you can make up for the lost time. You can rely on Magic FinServ to facilitate highly accurate and incisive forecasts by regulating the data pool. With our DCAM strategy and our bespoke tool - DeepSightTM , you can get better and better at predicting market outcomes and making timely adjustments.
Here's our DCAM strategy for it:
- Ensure data is clean and consolidated
- Use APIs and ensure that data is consolidated in one common source – key to our DCAM strategy
- Supplement structured data with alternative data sources
- Ensuring that data is available for slicing and dicing.
To conclude, the revenue and profits of the organization and associated customers depend on accurate predictions. And if predictions or forecasts go wrong, there is an unavoidable domino effect. Investors lose money, share value slumps, hiring freezes, people lose jobs, and willingness to trust the organization goes for a nosedive.
So, invest wisely and get your data in shape. For more information about what we do, email us at mail@magicfinserv.com